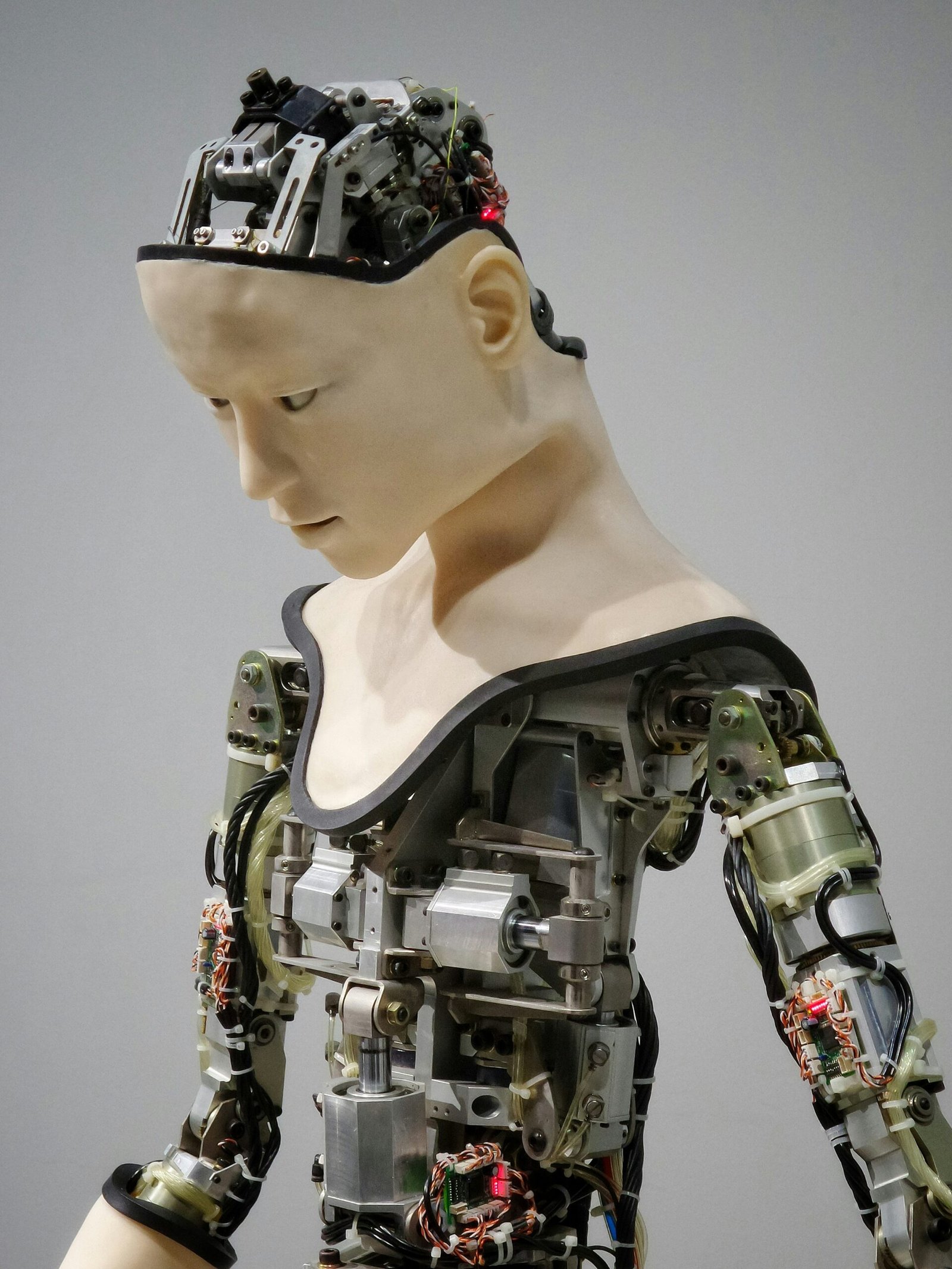
Introduction to Machine Learning in Healthcare
Machine learning, a subset of artificial intelligence (AI), involves algorithms that enable computers to learn from and make predictions based on data. In the healthcare domain, machine learning has emerged as a transformative force, offering innovative solutions to various challenges faced by medical professionals and institutions. The future of machine learning in healthcare is predicated on its ability to analyze vast amounts of patient data, identify patterns, and support clinical decision-making, ultimately leading to improved patient outcomes.
Currently, machine learning applications in healthcare range from diagnostic imaging to personalized medicine. For instance, algorithms can analyze medical images such as X-rays, MRIs, and CT scans, assisting radiologists in identifying anomalies with increased accuracy and speed. This not only enhances diagnostic precision but also reduces the burden on healthcare professionals. Additionally, machine learning models can predict disease outbreaks and track patient health trends, enabling timely interventions that can save lives.
Furthermore, machine learning applications extend to operational efficiencies in healthcare systems. By optimizing resource allocation, enhancing supply chain management, and predicting patient admission rates, healthcare organizations can improve their overall performance and reduce costs. The integration of machine learning into electronic health records (EHR) provides robust analytical tools that aid in identifying the best treatment options based on historical data, ensuring that personalized treatment plans are tailored to individual patients.
As healthcare continues to evolve, the significance of machine learning within the sector will grow. Its capability to unlock insights from complex datasets can lead to groundbreaking advancements not only in treatment methodologies but also in preventive care. As we delve deeper into the potential of machine learning in healthcare, it is essential to understand both its current applications and its future implications in revolutionizing diagnosis, treatment, and patient care.
Enhancing Diagnostics with Machine Learning
Machine learning has emerged as a potent tool in the quest to enhance diagnostics in healthcare. By leveraging advanced algorithms, healthcare professionals are increasingly able to improve diagnostic accuracy and speed. One of the most notable applications of machine learning in this context is the use of image recognition technology in radiology. Here, machine learning algorithms analyze medical images, such as X-rays, MRIs, and CT scans, identifying abnormalities that may not be easily discernible to the human eye. This not only aids radiologists in diagnosing conditions earlier but also significantly reduces the chances of misdiagnosis.
In addition to image recognition, predictive analytics powered by machine learning is becoming essential for managing chronic diseases. By sorting through vast amounts of patient data, machine learning algorithms can identify patterns that suggest the likelihood of disease progression. For instance, these predictive models can assess risk factors and suggest individualized treatment plans for patients battling conditions like diabetes or heart disease. Such tailored approaches, enabled by the future of machine learning in healthcare, allow physicians to intervene proactively, potentially preventing complications before they arise.
Real-world case studies underline the transformative impact of machine learning in diagnostics. For instance, a prominent healthcare institution successfully implemented a machine learning algorithm to analyze radiological images, resulting in a 20% increase in diagnostic accuracy for lung cancer detection. Similarly, another study showcased the effectiveness of predictive analytics in identifying patients at risk for heart failure, allowing for timely interventions and better patient outcomes. These tangible successes indicate a promising future where machine learning continues to elevate diagnostic precision, ultimately fostering a more proactive healthcare environment.
Personalized Treatment Plans Through Data Analysis
The future of machine learning in healthcare is significantly shaping personalized treatment plans, a process that encompasses the analysis of vast amounts of individual patient data. As healthcare evolves, the ability to tailor treatments to the unique genetic makeup, lifestyle factors, and medical history of each patient becomes increasingly crucial. Machine learning algorithms analyze these diverse data sets to identify patterns and predict patient responses to various treatment options, thereby enhancing the precision of care.
Genetic information plays a pivotal role in this paradigm shift. Utilizing genomic sequencing, healthcare professionals can better understand how specific genetic variations influence a patient’s response to medications. Machine learning applications facilitate the integration of this data, enabling clinicians to prescribe targeted therapies that are more likely to succeed based on the patient’s genetic profile. By unlocking the complexities of genetics, machine learning empowers healthcare providers to optimize treatment strategies, reducing trial-and-error approaches traditionally associated with prescribing.
Beyond genetics, lifestyle factors also contribute to the development of personalized treatment plans. Machine learning tools can analyze data from wearable devices and health applications, providing insights into patients’ daily activities, dietary habits, and overall health metrics. This comprehensive approach allows healthcare providers to design interventions tailored not only to the clinical symptoms of a patient but also to their personal circumstances. For instance, a treatment plan for managing diabetes may incorporate dietary recommendations that resonate with the patient’s preferences and lifestyle, thereby enhancing adherence and effectiveness.
Moreover, a patient’s treatment history is critically evaluated to inform future decisions. Machine learning models can ascertain successful past treatments and predict future outcomes, allowing for continuous refinement of care strategies. The integration of such sophisticated technology means that personalized healthcare is no longer a distant possibility but a present reality, fundamentally transforming how care is delivered and experienced.
Machine Learning in Drug Discovery and Development
The integration of machine learning in drug discovery and development is transforming the healthcare landscape by streamlining processes that were once cumbersome and time-consuming. Traditional drug discovery can take over a decade, with significant financial investments at each stage. However, the application of advanced algorithms allows for more efficient predictions of molecular behavior, which significantly accelerates the identification of potential drug candidates. Using large datasets, machine learning models can analyze biochemical interactions and predict the efficacy of compounds, thus enhancing the initial stages of drug development.
One of the major advancements in this realm is the ability of machine learning algorithms to dissect vast amounts of experimental data to identify biomarkers associated with therapeutic responses. By leveraging these insights, researchers can tailor drug development to individual patient profiles, known as personalized medicine, thus improving the likelihood of clinical success. For example, companies like Atomwise utilize convolutional neural networks to screen millions of potential drug compounds rapidly, resulting in the identification of promising candidates that may have gone unnoticed in traditional methods.
Moreover, machine learning plays a crucial role in refining clinical trial designs. By employing predictive analytics, researchers can optimize trial parameters before they commence, thereby identifying potential challenges early and minimizing dropout rates. This not only accelerates the trials but also enhances patient safety and ensures regulatory compliance. The success seen in trials for complex diseases such as cancer and neurodegenerative disorders illustrates how machine learning strategies can address previously insurmountable bottlenecks in drug development.
In conclusion, the future of machine learning in healthcare, particularly in drug discovery and development, promises to reduce costs, improve the efficiency of processes, and ultimately lead to the timely availability of new therapeutic options for patients. As these technologies evolve, they will undoubtedly play a pivotal role in shaping innovative approaches to healthcare solutions.
Predictive Analytics for Preventive Health
Machine learning has emerged as a transformative force in the healthcare sector, particularly in the realm of predictive analytics for preventive health. By harnessing vast amounts of data, healthcare providers can foresee potential health issues, enabling timely interventions and more efficient population management. The future of machine learning in healthcare is increasingly intertwined with the proactive approach of predicting health events before they escalate into serious conditions.
One of the critical applications of predictive analytics lies in identifying patients at risk for chronic diseases. For example, algorithms can analyze electronic health records (EHRs) and additional patient data to predict the likelihood of developing diseases such as diabetes or heart disease. These predictive models utilize various factors, including demographics, medical history, and lifestyle choices, to generate risk scores that can guide clinical decisions. Consequently, physicians can implement personalized wellness plans that focus on preventive measures tailored to individual patients, reflecting the future of machine learning in healthcare.
Moreover, successful examples of predictive models are already making a significant impact. For instance, organizations have developed machine learning systems that analyze data from wearable devices, allowing for real-time monitoring of patient health metrics. This proactive approach facilitates early interventions, helping to reduce hospital admissions and improve patient outcomes. Additionally, predictive analytics is being implemented in population health management, where healthcare providers can identify trends and manage resources efficiently across demographics, ensuring that interventions target those most in need.
As the landscape of healthcare continues to evolve, the application of machine learning and predictive analytics will play a pivotal role in shaping preventive health strategies. By leveraging data effectively, healthcare professionals can not only improve individual patient care but also enhance the overall health of communities, paving the way for a more sustainable and efficient healthcare system in the future.
Challenges and Ethical Considerations
The future of machine learning in healthcare presents an array of challenges and ethical considerations that must be addressed to harness its full potential effectively. One of the dominant issues surrounding the implementation of machine learning technologies is data privacy. The sensitive nature of healthcare data necessitates stringent measures to protect patient information from unauthorized access and misuse. As machine learning models are trained on vast amounts of data to improve accuracy in diagnosis and treatment recommendations, ensuring that personal health information remains confidential is paramount. This challenge compels healthcare organizations to adopt robust data governance frameworks that prioritize patient privacy while still leveraging advanced analytics.
Another critical concern is algorithm bias. Machine learning algorithms are only as good as the data they are trained on, which means that any bias present in training data can lead to skewed outcomes. For instance, if a model is predominantly trained on data from one demographic group, it may not perform well for others, resulting in unequal access to care or misdiagnosis. The future of machine learning in healthcare hinges on developing strategies to identify and mitigate algorithm bias, reflecting the need for diverse and representative data sets in model training. Additionally, continuous monitoring and adjusting of algorithms are essential to ensure fair treatment across all patient groups.
Furthermore, the establishment of regulatory frameworks is crucial in the integration of machine learning tools in healthcare settings. These regulations should govern not only the use of algorithms but also the transparency in their decision-making processes. Stakeholders, including policymakers, healthcare providers, and technology developers, must collaborate to create standards that promote accountability and provide guidelines for ethical practices. Remaining proactive in addressing these challenges is vital to maintain trust among patients and ensure that machine learning enhances healthcare delivery equitably and responsibly.
Future Trends in Machine Learning for Healthcare
The future of machine learning in healthcare is poised for significant advancements that will reshape various aspects of the industry. One prominent trend is the enhancement of artificial intelligence algorithms, which are becoming increasingly sophisticated. These algorithms are expected to offer improved diagnostic capabilities and predictive analytics, allowing healthcare providers to deliver more accurate and timely interventions. For example, advancements in deep learning techniques can facilitate the analysis of large datasets from electronic health records, leading to better-informed decision-making processes in clinical settings.
Additionally, the integration of machine learning with the Internet of Things (IoT) is anticipated to revolutionize patient monitoring and care. By harnessing data from wearable devices and connected medical equipment, machine learning algorithms can analyze real-time health information to identify potential issues before they escalate. This proactive approach not only improves patient outcomes but also enhances the efficiency of healthcare delivery systems by reducing unnecessary hospital visits and interventions.
Another promising development on the horizon is the incorporation of telemedicine in conjunction with machine learning technologies. The combination of virtual healthcare services and advanced analytical tools can broaden access to care, especially for patients in remote areas. By utilizing machine learning to analyze patient data gathered through telemedicine platforms, healthcare providers can tailor treatment plans more effectively, ensuring that patients receive personalized care based on their unique health profiles.
Overall, as we look towards the next decade, the future of machine learning in healthcare will undoubtedly lead to innovative solutions that streamline processes, enhance patient experiences, and ultimately improve health outcomes. Through the continuous evolution of AI algorithms, the integration of IoT, and the expansion of telemedicine, the healthcare landscape will transform, making care more accessible and efficient for patients worldwide.
Real-World Applications and Case Studies
Numerous organizations have begun to harness the power of machine learning in healthcare, resulting in significant advancements in diagnosis, treatment, and patient care. One notable example is the use of machine learning algorithms at the Mount Sinai Health System in New York. Researchers developed predictive models that analyze electronic health records to identify high-risk patients for various conditions, including heart disease and diabetes. By implementing these models, the organization has achieved a reduction in emergency room visits and improved the management of chronic diseases, showcasing the potential of the future of machine learning in healthcare.
Another compelling case study is that of Stanford University’s research team, which developed an innovative deep learning algorithm to detect pneumonia in chest X-rays. The algorithm performs at a level comparable to human radiologists, effectively increasing diagnostic accuracy. This implementation not only streamlines the radiological assessment process but also underscores the capabilities of machine learning in enhancing diagnostic tools. Such advancements pave the way for improving patient outcomes and ensuring timely treatment.
Furthermore, a multi-national pharmaceutical company, Novartis, has successfully integrated machine learning into its drug discovery process. By analyzing vast datasets, the company has been able to identify potential drug candidates at an unprecedented speed, significantly reducing the time from initial discovery to clinical trials. This application highlights how the future of machine learning in healthcare is revolutionizing research and development, enabling faster access to effective medications.
Ultimately, the lessons learned from these implementations emphasize the importance of collaboration between healthcare professionals, data scientists, and technology experts to fully realize the potential of machine learning tools. These case studies illustrate not only successful applications but also the ongoing journey of integrating machine learning into the healthcare landscape.
Conclusion: Embracing the Future of Healthcare
As we navigate the evolving landscape of healthcare, it is evident that the future of machine learning in healthcare holds transformative potential. The integration of advanced algorithms and data-driven insights is set to enhance diagnosis, streamline treatment processes, and ultimately improve patient care. By leveraging the vast amounts of data generated in healthcare settings, machine learning can identify patterns and anomalies that may be imperceptible to human practitioners, paving the way for more accurate and timely diagnoses.
Moreover, machine learning technologies not only focus on predictive analytics but also extend to personalized treatment plans. Tailoring therapies based on individual patient data ensures more effective healthcare strategies that prioritize patient outcomes. The implementation of these machine learning systems can lead to significant efficiencies, reducing healthcare costs and freeing up valuable resources for providers, which can be redirected towards patient care.
However, the successful adoption of machine learning in healthcare is contingent upon ongoing collaboration between technology experts and healthcare professionals. This partnership is essential for addressing challenges such as data privacy, ethical considerations, and integration with existing systems. By fostering a culture of communication and education, both sectors can cultivate an environment where innovative solutions thrive, ultimately advancing the quality of care delivered to patients.
In conclusion, embracing the future of machine learning in healthcare is not merely an option; it is a necessity for progress. Stakeholders must remain proactive in exploring the capabilities of these technologies while ensuring they are implemented responsibly and ethically. The potential benefits are immense, and with a commitment to collaboration and innovation, the future looks promising for both healthcare providers and patients alike.