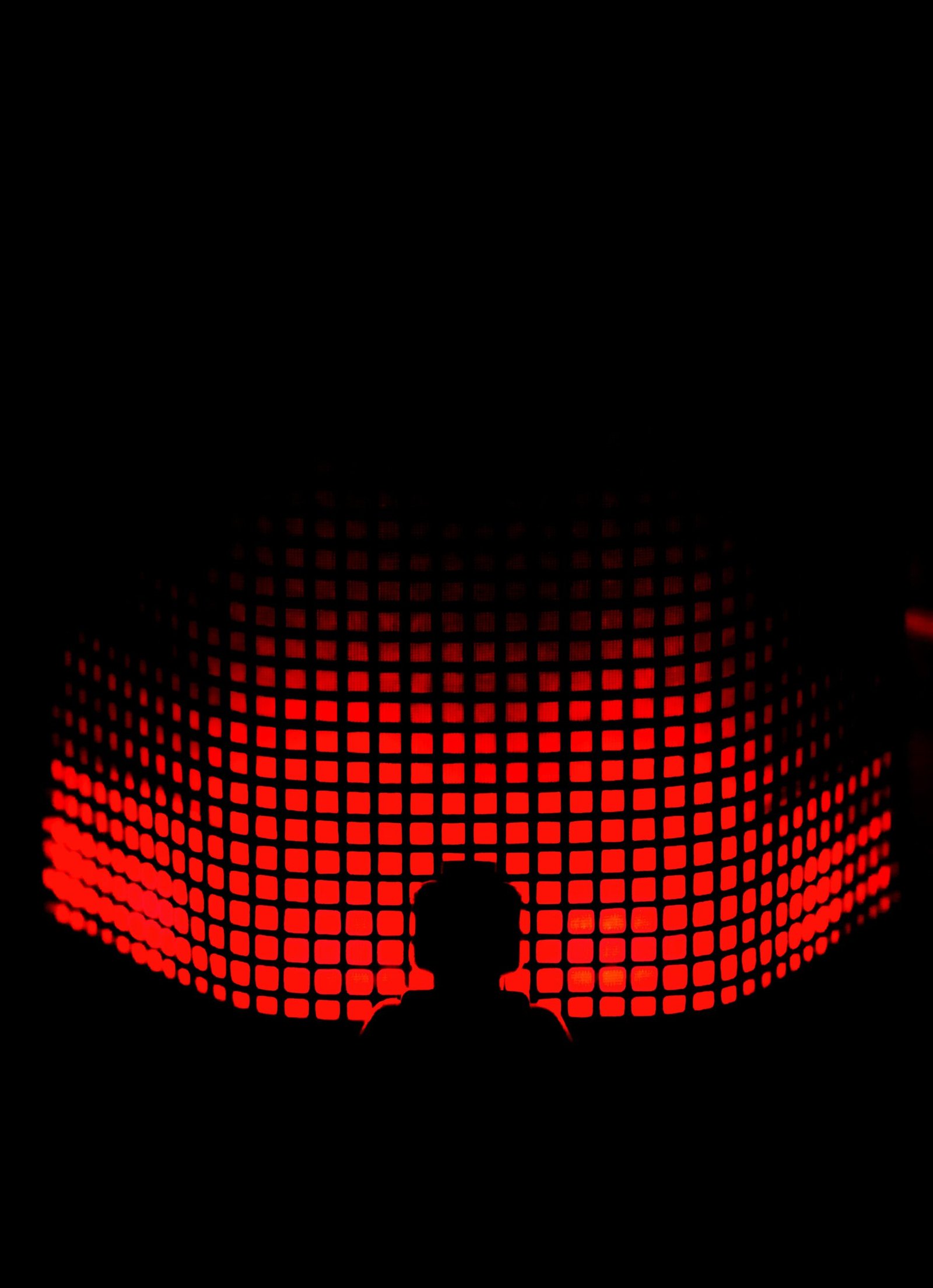
Introduction to Machine Learning in Healthcare
Machine learning, a subset of artificial intelligence (AI), refers to the development of algorithms that enable computers to learn from and make predictions based on data. This technology has gained significant traction across various sectors, particularly in healthcare, where it promises to transform the landscape of medical practice. As healthcare becomes increasingly data-driven, the applications of machine learning emerge as a pivotal component in analyzing vast amounts of information swiftly and accurately.
The significance of machine learning in healthcare has grown due to its capacity to process complex datasets and extract valuable insights. Techniques such as supervised learning, unsupervised learning, and reinforcement learning are being utilized to advance various healthcare applications. Supervised learning, for instance, involves training algorithms on labeled datasets, allowing for predictions in diagnostic procedures. In contrast, unsupervised learning helps in uncovering hidden patterns within patient data, which can lead to better understanding disease progression and treatment responses.
The evolution of technology in the healthcare sector has driven the integration of machine learning into various solutions. With the digitization of health records and the proliferation of wearable devices, the healthcare ecosystem is generating immense volumes of data. Consequently, healthcare professionals are motivated to harness the capabilities of machine learning to enhance patient care, streamline operations, and improve clinical outcomes. The predictive analytics offered by machine learning can aid in early diagnosis, personalized treatment plans, and efficient resource allocation, ultimately benefiting both patients and healthcare providers.
As we continue to witness advancements in machine learning, the potential applications in healthcare remain vast and largely untapped. This technology serves not only to augment existing medical practices but also to pave the way for innovative approaches that could redefine patient care in the years to come.
Predictive Analytics for Patient Outcomes
Machine learning has revolutionized the healthcare sector, particularly through the implementation of predictive analytics aimed at improving patient outcomes. By harnessing large datasets from electronic health records and other sources, machine learning algorithms can identify patterns and trends that may not be immediately apparent to healthcare professionals. This advanced analytical capability enables the prediction of various patient-related outcomes, such as complications, readmission risks, and treatment responses.
Through sophisticated models, predictive analytics can estimate the likelihood of adverse events occurring in patients, allowing for timely interventions that can mitigate risks. For instance, machine learning models assess a range of data points, including demographic information, medical history, and current health status, to forecast the potential for hospital readmission. Such proactive identification equips healthcare providers with the necessary insights to develop tailored care plans, ultimately enhancing patient satisfaction and reducing overall healthcare costs.
One notable case study showcasing the applications of machine learning in predictive analytics involves a hospital that implemented a machine learning model to predict sepsis in patients admitted to the emergency department. By analyzing vital sign data and laboratory results, the model successfully identified at-risk patients, allowing for early treatment and significantly decreasing mortality rates associated with sepsis.
Moreover, another application includes predicting patient responses to specific treatments based on historical data. By evaluating past treatment outcomes, machine learning can assist healthcare professionals in selecting the most effective therapies for individual patients. This personalized approach fosters better adherence to treatment protocols and optimizes health outcomes.
In conclusion, the integration of predictive analytics powered by machine learning into healthcare practices significantly enhances patient outcomes. By providing actionable insights based on comprehensive data analysis, these applications not only improve the quality of care but also pave the way for innovative practices in healthcare delivery.
Personalized Medicine and Treatment Plans
Machine learning has emerged as a transformative force in the realm of personalized medicine and treatment planning. Traditionally, treatment protocols have followed a one-size-fits-all approach, which may not consider the unique genetic makeup and lifestyle factors of individual patients. However, with the advent of machine learning technologies, healthcare professionals can analyze vast amounts of data, including genetic sequences, environmental influences, and patient lifestyle choices, to tailor treatment plans that fit the specific needs of each patient more accurately.
One significant application of machine learning lies in its ability to process complex datasets quickly and efficiently. Algorithms can identify patterns and correlations that might not be readily apparent to human analysts. For instance, in oncology, machine learning models can analyze genetic mutations and predict how individual patients will respond to specific cancer therapies. A study from a leading medical institute has shown that patients whose treatment plans were guided by machine learning algorithms experienced improved outcomes, resulting in higher response rates to therapies and reduced side effects.
Additionally, personalized medicine extends beyond genetic factors. Machine learning also evaluates environmental and lifestyle-related data, such as diet, exercise habits, and psychosocial factors, to refine treatment approaches. For example, wearable technology that tracks patient activity levels can provide input for machine learning models to recommend precise interventions that can lead to better health outcomes. As a result, patients report increased satisfaction due to the enhanced effectiveness of customized treatment plans and the proactive nature of their healthcare management.
Overall, the applications of machine learning in personalized medicine signify a shift towards more individualized patient care. By harnessing the power of advanced algorithms to analyze comprehensive data, healthcare providers can craft optimal treatment strategies that cater uniquely to each patient.
Diagnosis and Disease Detection
Machine learning has emerged as a transformative force within healthcare, particularly in the realms of diagnosis and disease detection. The advent of advanced algorithms has unlocked capabilities that enhance the accuracy and efficiency of identifying medical conditions. One of the most significant applications of machine learning can be seen in image recognition technologies, which are now pivotal in radiology and pathology. These algorithms analyze medical imaging data—such as MRIs, CT scans, and X-rays—enabling healthcare professionals to detect anomalies that may indicate diseases with remarkable precision.
For instance, convolutional neural networks (CNNs) are frequently employed in radiology to facilitate the interpretation of images. These sophisticated models have been trained on vast datasets, allowing them to learn patterns associated with various diseases. As a result, machine learning applications yield rapid and reliable diagnoses, significantly reducing the time required to evaluate imaging data and potentially improving patient outcomes through earlier intervention.
Beyond imaging, machine learning is enhancing the diagnosis process through the analysis of medical records. Algorithms are now capable of sifting through extensive datasets, identifying relevant health indicators and patterns that could signify the onset of specific diseases. By employing natural language processing techniques, these tools interpret unstructured data from clinical notes and patient histories, further refining the diagnostic process based on a comprehensive understanding of individual patient cases.
The applications of machine learning in diagnosing diseases have already demonstrated effectiveness in various real-world settings. Studies indicate that early detection facilitated by these technologies markedly increases the chances of successful treatment. Consequently, the integration of machine learning into healthcare systems represents a significant leap forward, making diagnoses more accurate and timely, ultimately improving the standard of care available to patients.
Enhancing Medical Imaging with AI
In the realm of healthcare, the applications of machine learning are making significant strides, particularly in the field of medical imaging. Medical imaging techniques, such as X-rays, MRIs, and CT scans, play a pivotal role in diagnosing various conditions. The integration of artificial intelligence (AI) through sophisticated machine learning algorithms has transformed how healthcare professionals interpret these images, resulting in increased accuracy and efficiency in diagnosis.
Machine learning models are being developed to analyze vast datasets of imaging studies. These models can identify patterns that may not be easily visible to the human eye, allowing for early detection of anomalies or diseases, such as tumors or fractures. For instance, in radiology, AI algorithms have been trained to distinguish between malignant and benign lesions in mammograms with a level of accuracy that rivals or surpasses that of human radiologists. This not only aids in timely diagnosis but also reduces the cognitive load on healthcare professionals, enabling them to focus on more complex cases.
Moreover, the speed at which these AI systems can process and interpret medical images significantly enhances overall workflow in clinical settings. For example, in emergency departments where time-sensitive decisions are crucial, machine learning can rapidly evaluate CT scans to identify critical conditions such as strokes or pulmonary embolisms. By providing healthcare providers with quick and reliable insights, the applications of machine learning are facilitating a more rigorous and responsive approach to patient care.
Several hospitals and medical institutions have already implemented machine learning-driven solutions, witnessing remarkable improvements in diagnostic accuracy and workflow efficiency. These advancements underscore a promising future where AI complements human expertise, ultimately leading to better patient outcomes and revolutionizing the standards of medical imaging.
Robotics and Autonomous Systems in Surgery
The integration of machine learning in the field of robotics and autonomous systems has brought significant advancements to surgical practices. Robotic surgery systems, augmented with artificial intelligence (AI), exemplify the applications of machine learning that enhance surgical capabilities. These systems, such as the Da Vinci Surgical System, utilize precise robotic arms controlled by surgeons, allowing for minimally invasive procedures that lead to improved patient outcomes.
Machine learning algorithms enable these robotic systems to learn from vast amounts of surgical data, improving their accuracy and effectiveness over time. For instance, these algorithms can analyze surgical videos and instrument movements, identifying optimal techniques and suggesting enhancements to surgical methods. By supporting informed decision-making, AI-assisted tools contribute to better precision during complex operations, thus reducing the risk of complications.
One notable application of machine learning in surgery is in the planning and execution of procedures. Surgeons can leverage predictive analytics to assess patient-specific factors and health metrics, allowing for tailored surgical approaches based on individual needs. This personalized approach not only improves surgical outcomes but also minimizes recovery times, enabling patients to return to their daily lives more rapidly. Additionally, autonomous systems equipped with machine learning capabilities can monitor vital signs in real-time, adjusting surgical parameters as needed and ensuring a higher level of safety throughout the procedure.
Recent implementations of robotic surgery demonstrate these advancements effectively. For example, the use of robotic systems in prostatectomies has led to quicker recovery and less postoperative pain compared to traditional methods. Such cases highlight the substantial impact that machine learning-infused robotics and autonomous systems have on modern surgery. As technology evolves, the applications of machine learning in this domain are expected to expand, further revolutionizing healthcare and improving patient care.
Managing Healthcare Operations and Administration
Machine learning has emerged as a transformative force in the healthcare sector, particularly in enhancing operations and administrative tasks. By employing advanced algorithms and statistical models, healthcare facilities can harness the power of data to improve decision-making processes in several critical areas, including resource allocation and scheduling.
One of the significant applications of machine learning lies in optimizing resource allocation. Hospitals often face challenges related to staff shortages or excess capacity, which can lead to increased costs and decreased patient satisfaction. Machine learning algorithms can analyze vast datasets that include historical admission rates, staffing schedules, and patient demand forecasts. By predicting when resources will be needed most, administrators can allocate staff and equipment more effectively, thereby enhancing operational efficiency and reducing wait times for patients.
Similarly, machine learning can significantly improve scheduling systems, ensuring that healthcare professionals are available when they are most needed. For instance, predictive analytics can assess appointment trends and patient no-show rates, allowing healthcare providers to create more accurate schedules. Improved scheduling not only optimizes the use of medical staff but also enhances patient flow through healthcare facilities, ultimately leading to better service delivery and increased patient satisfaction.
The impact of machine learning on healthcare operations extends to administrative efficiencies as well. Routine tasks, such as patient registration, billing, and insurance verification, can be streamlined using machine learning applications. By automating these processes, healthcare organizations can reduce administrative costs and minimize the likelihood of errors, which not only saves time but also contributes to improved patient experiences.
As machine learning continues to evolve, its applications in managing healthcare operations and administration will likely expand, fostering an environment that prioritizes efficiency, cost-effectiveness, and superior service delivery.
Ethical Considerations of Machine Learning in Healthcare
The implementation of machine learning in healthcare brings forth numerous ethical considerations that merit careful attention. As the applications of machine learning become increasingly prevalent, healthcare institutions must grapple with the significant implications surrounding data privacy. Patient data is often sensitive and personal; therefore, maintaining confidentiality while utilizing this information for training algorithms is crucial. Organizations need to establish robust data governance frameworks to ensure that personal data is accessed, stored, and utilized in ways that protect patient identities and comply with regulatory standards.
Another critical ethical concern is the potential for bias in algorithm design. If the data used to train machine learning models is not representative of the diverse patient population, the resulting algorithms may exhibit bias, leading to inequitable healthcare outcomes. This could disproportionately affect marginalized groups, exacerbating existing health disparities. Therefore, it is imperative to employ diverse datasets and adopt inclusive practices during the development and validation of machine learning algorithms. Continuous monitoring and adjustment of these models are essential to mitigate biases and ensure fairness in healthcare delivery.
Moreover, while machine learning can enhance decision-making processes in healthcare, it is essential to maintain human oversight. Medical professionals should not solely rely on algorithmic outputs without applying their clinical judgment. Machine learning applications should serve as decision-support tools rather than replacements for human expertise. This balance is essential to preserve the ethical standards of care, ensuring that healthcare practitioners remain accountable for their decisions and the wellbeing of their patients.
Ultimately, the integration of ethical guidelines into the applications of machine learning will facilitate responsible and equitable healthcare practices. Stakeholders, including policymakers, healthcare providers, and technologists, must collaborate to establish these standards, ensuring that the benefits of machine learning are realized without compromising ethical principles.
Future Trends in Machine Learning and Healthcare
The intersection of machine learning and healthcare is set to undergo significant transformations in the coming years, with several trends anticipated to shape the industry. As technology continues to evolve, the applications of machine learning will become increasingly sophisticated, leading to enhanced patient care and operational efficiency within healthcare systems. One notable trend is the growing use of predictive analytics. By leveraging vast amounts of data, healthcare providers will be able to foresee patient outcomes more accurately, allowing for proactive interventions that can mitigate risks and improve health trajectories.
Another important trend is the rise of personalized medicine, which utilizes machine learning algorithms to tailor treatments to individual patients based on their unique genetic makeup and health histories. This approach not only has the potential to improve treatment efficacy but also minimizes adverse effects, thus revolutionizing how diseases are managed. Additionally, the integration of machine learning with wearable health technologies will provide real-time health monitoring, giving healthcare professionals valuable insights into patients’ conditions and facilitating timely responses to health issues.
Moreover, advancements in natural language processing (NLP) are expected to greatly enhance the ability to analyze unstructured data, such as clinical notes and research publications. This will lead to more informed clinical decision-making and streamlined administrative processes. It is also likely that we will see an increase in collaborative systems where machine learning applications work alongside healthcare professionals, providing them with data-driven insights that support their expertise.
The impact of these innovations is profound, as they promise to enhance the overall effectiveness and efficiency of healthcare delivery. Continuous development and adaptation in machine learning will be essential to keeping pace with the dynamic needs of healthcare environments, ensuring that patient care systems improve and evolve to meet future demands.